Evidence-based assessment/Step 3: Evaluate risk and protective factors and moderators
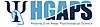
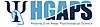
HGAPS is finding new ways to make psychological science conferences more accessible!
Here are examples from APA 2022 and the JCCAP Future Directions Forum. Coming soon... ABCT!
~ More at HGAPS.org ~
Medical disclaimer: This page is for educational and informational purposes only and may not be construed as medical advice. The information is not intended to replace medical advice offered by physicians. Please refer to the full text of the Wikiversity medical disclaimer. |
EBA Implementation |
---|
Assessment phases |
Steps 1-2: Preparation phase |
Steps 3-5: Prediction phase |
Steps 6-9: Prescription phase |
Steps 10-12: Process/progress/outcome phase |
Prediction: Risk and Protective Factors[edit | edit source]
Overview[edit | edit source]
The goal here is to find clinically relevant risk factors (things that increase the probability of a particular problem) and protective factors. This is hard to do in practice, at least with any consistency. To make it more manageable, we will build lists, try to identify which factors are especially potent, and start to build checklists to make sure that we remember to ask about at least these (with a nod towards Atul Gawande and The Checklist Manifesto). We also walk through how one could integrate research about risk and protective factors into an algorithm to update the probability about a particular issue for a given client.
Rationale[edit | edit source]
Description of Risk Factors[edit | edit source]
Risk factors could be related to suicidal ideation, suicidal behavior, or non-suicidal self injury (here is a page with information about publicly available data sets with some basic information about these variables).
Protective Factors[edit | edit source]
Intelligence, higher SES, higher self-esteem,having a supportive adult, and positive peer relationships are all examples of protective factors that are likely to buffer against the effects of risk factors, and improve treatment response and outcome if problems arise. These protective factors are general (non-specific) and convey protection across a wide range of issues. Future research could start to quantify the effect size for different conditions. Having a supportive adult may have different sized effects on externalizing problems versus anxiety or a psychotic disorder, for example.
Ranking the Effect Size of Factors[edit | edit source]
Biggest Factors: These are ones with effect sizes so large that they are likely to have an impact on the decision-making process for an individual client. In the Prediction context, these are ones that are likely to have diagnostic likelihood ratios (DLRs) (link) of 5 or larger. (A DLR of 10 is "large" for a test result according to Straus and colleagues, but there are few risk factors we have seen that reach this range. DLR of 5+ is big enough that we definitely would want to include it in the assessment and decision-making process).
Moderate Factors: DLRs of 2 or larger (though less than 5, as above).
Small Factors: Statistically significant but with an effect size corresponding to a DLR < 2, or equivocal results. Usually not large enough to inform decision making at the individual level.
Add links to DLR, nomogram, decision-making, EBM resources
Steps to put into practice[edit | edit source]
There are several options about how to use information about risk and protective factors clinically.
The simplest is to just be sure to ask about them and write down which are present. Making a checklist of common or important ones would improve the consistency of asking about them. The DSM often includes notes about checking for alternate explanations, such as substance intoxication or effects of a general medical condition, before making a diagnosis. As psychologists, we typically do not get a lot of formal training about the effects of medications or street drugs, nor do we get much guidance about common medical conditions that could lead to psychological symptoms. Talking with a pediatrician could be a way of getting more of a sense of what some key issues might be.
One step further in terms of sophistication would be to rank the risk and protective factors in terms of effect size, as suggested in the section above. Research articles in psychology tend to use Pearson's correlation (r) or Cohen's d (a standardized mean difference); but studies of risk factors may report chi-squared analyses (with Cohen's w as a corresponding effect size, or a phi correlation) or odds ratios. As a rule of thumb, large effect sizes are going to always be clinically informative. Medium effect sizes are also helpful, but it is harder to know how much weight to give them in individual cases. Small effect sizes are usually not going to change things much on average for an individual client.
Even more helpful ways of incorporating risk and protective factors into clinical decision-making include using a risk calculator or a probability nomogram to combine the prior probability of a diagnosis or outcome with the change in the likelihood due to the risk/protective factor. The diagnostic likelihood ratio (DLR) is the most useful effect size for this purpose.
Machine learning models are starting to combine sets of risk and protective factors to make predictions about diagnoses and outcomes, too. Machine learning would have major advantages in terms of handling complex prediction models (i.e., lots of variables with different weights) and could reduce or eliminate the analytic burden; but these are not yet widely available clinically. The online calculators and probability nomograms are more practical for now.